The Intelligence of Loss in the Archive
Anna-Maria Meister and Rafael Uriarte
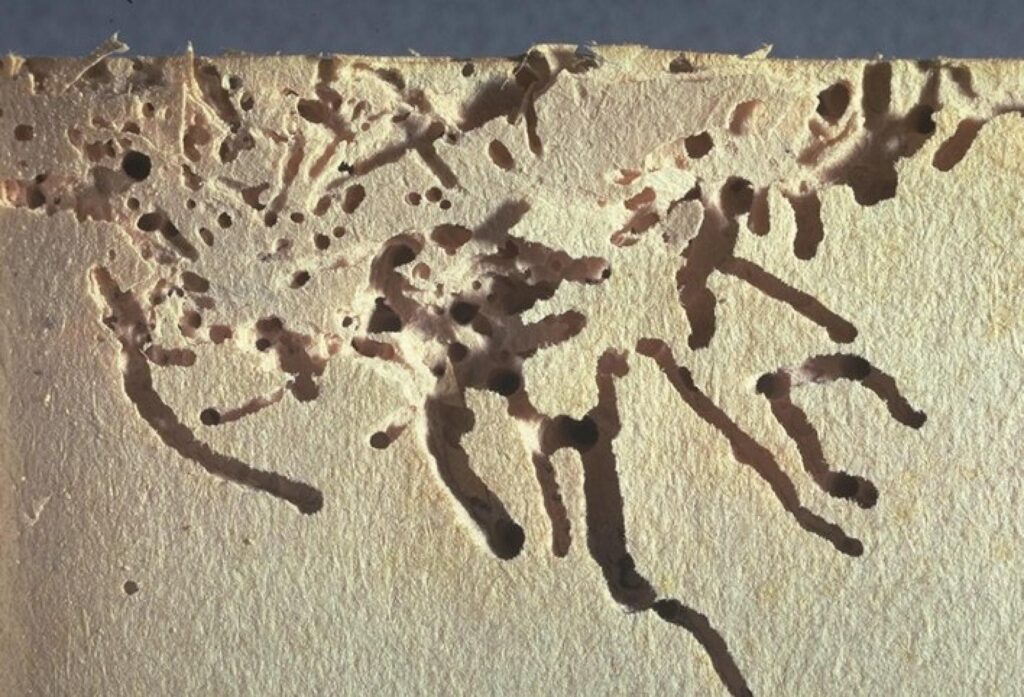
Archives are core sites of destruction, decay and loss. Whether through man-made destruction, be it intentional, arbitrary, or accidental—such as changes in institutional policy, forgetfulness, deliberate evidence destruction, wars, migration, or even the act of curating an exhibition—or so-called natural disasters such as earthquakes, fire or water have eradicated much of humanity’s stored matter, and keep doing so. From slowly dripping water under broken roofs or dropped bombs, archives are not “safe.” Small-scale destruction and micro damage aided by the long durée of the archival perspective can be just as devastating. Be it undetected or untreated mold, a colony of paper fish or simply crumbling paper, archives and their matter-data continuously disintegrate under human watch. And, not least, archives are full of things no-one can access, rendering things lost not due to their absence but their lack of retrievability within the archive itself: from vast collections hidden away in depot spaces forgotten for decades, or a misplaced model on a shelf, to digital born or digitized data stored on obsolete hardware, operated with deprecated software, defined with superseded or discontinued standards. Moving toward an age of “peak storage” (a limit not of technical expansion, but of sustainable storage where meaningful retrieval reaches its limits), we ask what can be learned through speculative destruction in the face of “peak storage,” mass migration and the predicted AI energy crisis?
The entropic nature of archives, where the very processes designed to preserve knowledge—whether on paper or servers—inevitably lead to transformation, selective preservation and loss. While in art and architecture history, narratives of loss and decay have started to play a more important role in historiography, AI is hailed as a promise against loss. But what if we treated forgetting not as a bug, but a feature? AI’s processes of erasure follow distinct patterns that may reveal new ways of managing archival sustainability. Its mechanisms of forgetting and loss—such as data selection, pruning, model drift, algorithmic obsolescence and capacity to (almost) randomize choices—offer alternatives to human-driven/caused archival destruction. Rather than treating AI as an optimized alternative for pattern recognition in archives, this research explores how AI’s patterns of forgetting might reshape our understanding of archival decay and persistence. By taking loss and erasure seriously—not as failures, but as integral to knowledge systems—, we try to explore how it might be an underappreciated field of exploration, one where its (at least seemingly) irrational and unpredictable human shape might be a form of intelligence AI could learn from.
Written from two perspectives, this essay approaches the intersection of archival disappearance and AI-driven erasure. What does the pattern of loss look like and can it be modelled? Reversely, can humans gain insights from AI-driven loss provide into archival decay? and? Investigating what is lost with which intentions, what is memorable only because it was lost, or how forgotten materials encorporate intelligence, this essay takes loss not as a problem, but as a promise of other ways of knowing.
The entropic nature of archives, where the very processes designed to preserve knowledge—whether on paper or servers—inevitably lead to transformation, selective preservation and loss. While in art and architecture history, narratives of loss and decay have started to play a more important role in historiography, AI is hailed as a promise against loss. But what if we treated forgetting not as a bug, but a feature? AI’s processes of erasure follow distinct patterns that may reveal new ways of managing archival sustainability. Its mechanisms of forgetting and loss—such as data selection, pruning, model drift, algorithmic obsolescence and capacity to (almost) randomize choices—offer alternatives to human-driven/caused archival destruction. Rather than treating AI as an optimized alternative for pattern recognition in archives, this research explores how AI’s patterns of forgetting might reshape our understanding of archival decay and persistence. By taking loss and erasure seriously—not as failures, but as integral to knowledge systems—, we try to explore how it might be an underappreciated field of exploration, one where its (at least seemingly) irrational and unpredictable human shape might be a form of intelligence AI could learn from.
Written from two perspectives, this essay approaches the intersection of archival disappearance and AI-driven erasure. What does the pattern of loss look like and can it be modelled? Reversely, can humans gain insights from AI-driven loss provide into archival decay? and? Investigating what is lost with which intentions, what is memorable only because it was lost, or how forgotten materials encorporate intelligence, this essay takes loss not as a problem, but as a promise of other ways of knowing.